What is RAG (Retrieval Augmented Generation)?
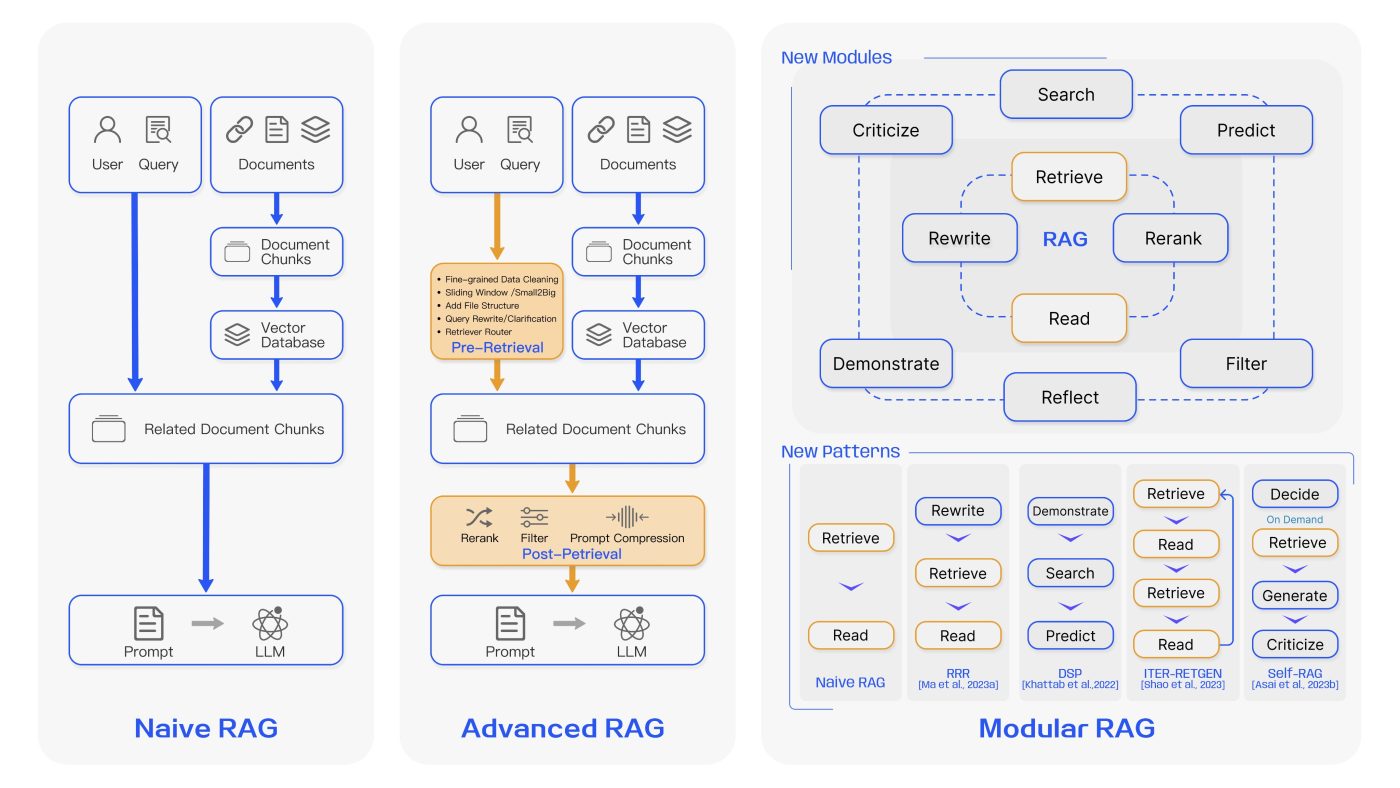
RAG (Retrieval-Augmented Generation) is an advanced AI architecture that combines the power of large language models (LLMs) with external knowledge retrieval. Here’s a breakdown of RAG, its workings, importance, and business applications:
You’re probably familiar with some of the shortcomings of current AI. For me, the most frustrating aspect is that you can’t rely on it for accurate information. Not only do current LLMs frequently ‘hallucinate’ facts, people, code libraries, events, and more – they state this information with such confidence that it can be hard to spot.
RAG is a hybrid AI system that enhances traditional language models by incorporating a retrieval step to fetch relevant information from external sources before generating responses. This approach allows AI to access up-to-date, factual information beyond its initial training data.
RAG not only makes AI more reliable, it introduces verifiability – simply put you can click on a link to the source and check it yourself. For example, Perplexity, a RAG application that also combines web search shows a list of sources at the top of the answer, as well as a numbered citation where it has written its response based on a specific source:
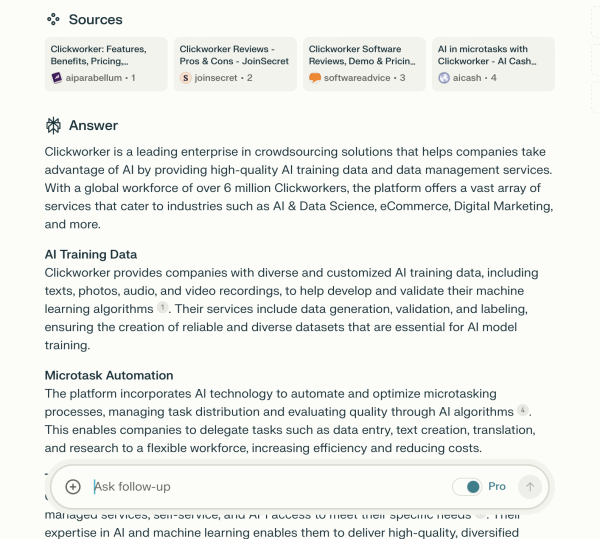
How RAG Works:
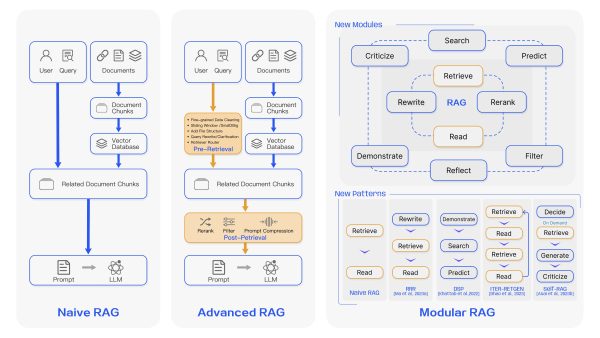
Image credit:Baoyu, Prompt Engineer
- Query Processing: The system receives a user query or prompt.
- Retrieval: It searches a knowledge base (e.g., documents, databases) for relevant information using vector search techniques.
- Augmentation: The retrieved information is combined with the original query.
- Generation: An LLM uses the augmented input to generate a response.
Why RAG is Important:
- Reduced Hallucinations: By grounding responses in retrieved facts, RAG minimizes AI hallucinations or fabrications.
- Up-to-date Information: RAG can access current data, overcoming the limitation of static training datasets.
- Customization: It allows integration of domain-specific or proprietary information.
- Improved Accuracy: Responses are more reliable and contextually relevant.
- Transparency: The system can cite sources, enhancing trustworthiness.
Top Applications in Business
- Customer Service: RAG-powered chatbots can provide accurate, context-aware responses, improving customer satisfaction and reducing support costs.
- Knowledge Management: Efficiently organize and retrieve company information, enhancing decision-making and productivity.
- Research and Development: Quickly access and synthesize relevant data from vast information repositories.
- Personalized Marketing: Create tailored content and recommendations based on up-to-date customer data and market trends.
- Legal and Compliance: Stay current with changing regulations and quickly retrieve relevant legal information.
- Training and Education: Develop adaptive learning systems that provide personalized, up-to-date educational content.
- Product Development: Integrate customer feedback and market data to inform product improvements and innovations.
- Financial Analysis: Combine historical data with current market information for more accurate forecasting and risk assessment.
By leveraging RAG, businesses can create more intelligent, adaptive, and trustworthy AI systems that drive growth through improved decision-making, enhanced customer experiences, and increased operational efficiency.
RAG-based AI assistantsare opening up new business opportunities by dramatically improving productivity and capabilities compared to traditional large language models (LLMs). RAG allows AI systems to access and leverage large knowledge bases and codebases to provide more accurate, contextual, and useful responses. This creates opportunities for companies to develop specialized AI assistants tailored to specific domains, industries, or enterprise environments.
Cursor AI is another RAG example, this time storing and retrieving a codebase as well as API and library documentation to provide the right context for LLMs to then write new code or edit existing parts of it:
One key business opportunity is in developing advanced context engines and retrieval systems. Having multiple “lenses” or context providers that can quickly pull relevant information from various sources is crucial for RAG performance. Companies that can build high-performance code search indexes, natural language search capabilities, and connectors to different data sources will be well-positioned in this space. There’s also potential for creating industry or domain-specific knowledge bases that can be used to augment general LLMs.
The shift towards agentic workflows enabled by RAG creates opportunities for workflow automation and productivity tools. As the article notes, iterative AI agents that can plan, execute subtasks, and refine their own work produce significantly better results than simple one-shot LLM responses. Businesses could develop specialized agents for tasks like research, coding, writing, or data analysis that leverage RAG to work more autonomously and produce higher quality output. There’s also potential for creating platforms that allow non-technical users to easily create and deploy custom AI agents for their specific needs.
Finally, the need for fast token generation in RAG systems opens up opportunities in AI infrastructure and model optimization. As highlighted, being able to quickly generate many tokens for internal agent reasoning is crucial for these iterative workflows. Companies that can provide high-performance, cost-effective infrastructure for running RAG systems at scale, or develop optimized models that balance speed and quality for RAG use cases, could find significant demand for their solutions as more businesses adopt these technologies.
Current Challenges of RAG
- Limited Contextual Understanding: Traditional RAG systems often struggle to grasp the nuances and overall context of a document corpus. They rely heavily on retrieved chunks or sub-documents, which can lead to a fragmented understanding of the information.
- Scalability Issues: As the document corpus grows, traditional RAG systems can become less efficient in retrieval processes. This is because they typically rely on vector similarity searches across all chunks, which can become computationally expensive and time-consuming for large datasets.
- Complexity in Integrating External Knowledge: Traditional RAG systems often find it challenging to meaningfully incorporate external knowledge sources into their retrieval and generation process. This limitation can result in responses that lack broader context or fail to connect related information from different sources.
- Lack of Relationship Understanding: RAG systems may miss important connections between different pieces of information, as they often treat chunks of text as independent units. This can lead to responses that fail to capture the interconnected nature of complex topics.
- Difficulty in Handling Multi-hop Questions: Questions that require information from multiple, indirectly related sources can be challenging for traditional RAG systems. They may struggle to connect the dots between different pieces of information that are not explicitly linked in the retrieved chunks.
- Limited Summarization Capabilities: Traditional RAG systems often struggle to provide summaries at varying levels of detail or abstraction, as they typically work with fixed-size chunks of text.
How GraphRAG Can Potentially Help
GraphRAG is a relatively new approach to RAG, using Knowledge Graphs to more effectively store and retrieve connected information. Knowledge Graphs have been used with great success, for example powering Google Search, so combining them with RAG feels like a natural progression.
- Enhanced Contextual Understanding: GraphRAG creates a knowledge graph that represents the entire document set with interconnected entities and relationships. This allows for a more comprehensive understanding of the context and themes present in the corpus, enabling more nuanced and contextually relevant responses.
- Improved Scalability: GraphRAG introduces a hierarchical community structure within the knowledge graph. This allows for more efficient retrieval by first identifying relevant communities and then drilling down to specific information, improving scalability for larger datasets.
- Easier Integration of External Knowledge: The knowledge graph structure of GraphRAG naturally allows for the integration of external knowledge by adding new nodes and relationships to the existing graph. This makes it easier to combine information from various sources in a coherent manner.
- Better Relationship Understanding: GraphRAG explicitly models relationships between entities through the knowledge graph structure. This allows the system to understand and utilize connections between different pieces of information, leading tomore insightful and contextually relevant responses.
- Improved Handling of Multi-hop Questions: The graph structure in GraphRAG allows for easier traversal of related information, making it more effective at answering complex, multi-hop questions by following paths in the knowledge graph.
- Multi-level Summarization: GraphRAG introduces a multi-level community structure (e.g., local, intermediate, and global levels) with summaries at each level. This allows for more flexible querying and summarization at different granularities of information.
- Better Source Attribution: GraphRAG maintains clear links between the knowledge graph nodes and the original source documents. This allows for better source attribution in the generated responses, enhancing transparency and trustworthiness.
While GraphRAG offers these significant improvements, it’s important to note that it comes with its own challenges, particularly in terms of computational cost and complexity. The process of creating and maintaining the knowledge graph, including entity extraction, relationship identification, and multi-level summarization, can be significantly more expensive than traditional RAG approaches. Therefore, while GraphRAG presents a promising solution to many RAG limitations, its implementation requires careful consideration of the trade-offs between improved performance and increased computational costs.
Performance Improvements with RAG
In a recent lecture from the Stanford CS25: Transformers United V3 course, Douwe Kiela from Contextual AI shared valuable insights on the current state and future of Retrieval-Augmented Generation (RAG) systems. His presentation highlighted several key areas where RAG is making significant strides and where future developments are likely to occur.
Kiela emphasized the substantial performance enhancements that RAG systems bring to language models:
- The ATLAS paper demonstrates significant improvements over closed-book models across various few-shot language modeling tasks.
- RAG systems can outperform much larger parametric models. For instance, the Retro paper showed that a 25x smaller retrieval-augmented model outperformed a larger language model in terms of perplexity.
Implementation Challenges
- Computational overhead: Updating document encoders is extremely expensive, requiring re-encoding of the entire index after each update.
- Latency issues: There’s a trade-off between cost and quality, implying that real-time retrieval can impact system responsiveness.
- Maintaining and updating knowledge bases: Various approaches to updating indices were discussed, including asynchronous updates and query-side only updates.
Ethical Considerations
Kiela touched on some ethical implications of RAG systems:
- Data provenance: RAG systems could potentially address legal concerns by training on “safe” data while accessing a broader, potentially riskier index at test time.
- Privacy: The lecture mentioned GDPR compliance as a motivation for RAG systems, as they allow for easier removal or revision of specific information.
RAG Variations and Enhancements
- Hybrid search combining sparse (BM25) and dense retrieval methods
- Multi-stage retrieval with re-ranking
- Active retrieval where the model learns when to retrieve
- Multimodal RAG incorporating vision (e.g., the Lens system for visual question answering)
Final Thoughts
Retrieval-Augmented Generation (RAG) represents a significant leap forward in AI technology, combining the power of large language models with the ability to access and utilize external knowledge sources. This hybrid approach addresses many limitations of traditional AI systems, offering improved accuracy, reduced hallucinations, and the ability to work with up-to-date information.
As we’ve explored, RAG systems have wide-ranging applications across various business sectors, from enhancing customer service to revolutionizing research and development processes. The technology’s ability to provide more contextually relevant and factually grounded responses opens up new possibilities for AI-driven solutions in knowledge management, personalized marketing, legal compliance, and beyond.
However, RAG is not without its challenges. Current systems face issues with scalability, contextual understanding, and the complexity of integrating diverse knowledge sources. Emerging solutions like GraphRAG show promise in addressing these limitations by leveraging knowledge graph structures to enhance contextual understanding and relationship mapping.
It’s now hard to imagine a future where some form of RAG technology is not a large part of daily life for millions of people. At the smallest scale, any knowledge worker can now have a truly personal AI assistant. And at the other end of the specturm, governments will have the ability to make more informed and effective decisions, taking advantage of the otherwise overwhelming amount of data they have access to.
For businesses and organizations looking to stay at the forefront of AI technology, understanding and leveraging RAG systems will be crucial. The potential for increased efficiency, improved decision-making, and enhanced user experiences makes RAG a key area to watch and invest in as we move forward in the age of AI-driven innovation.
Is AI on the Path to Superintelligence?
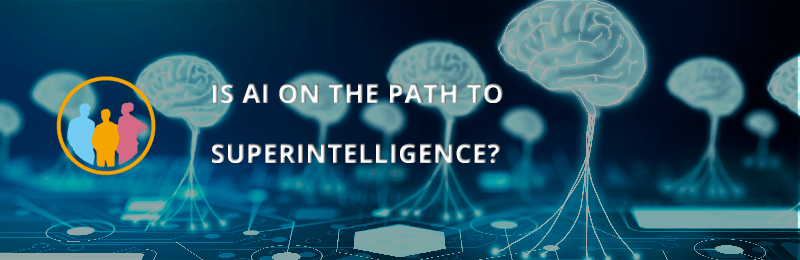
The rapid development in the field of artificial intelligence (AI) raises a crucial question: Will there ever be an AI superintelligence? The recent buzz around OpenAI and speculations about a mysterious project called “Q*” have reignited discussions about artificial general intelligence (AGI) and potential safeguards. Reports suggest that OpenAI has made progress in independently solving complex mathematical problems, which is seen as a step toward AGI. This has led to concerns and calls to slow down AI development and focus more on alignment with human values.
Regardless of specific advancements at OpenAI, the pace of AI development raises many fundamental questions. What is the current state of AGI research? What steps are necessary to get there? How do AGI and superintelligence differ? What ethical and societal implications arise from these developments? Experts shared their views and concerns on these topics during a virtual press briefing, emphasizing the importance of responsible and safe AI development.
Read moreA Milestone for Europe: The AI Act and Its Significance for Artificial Intelligence
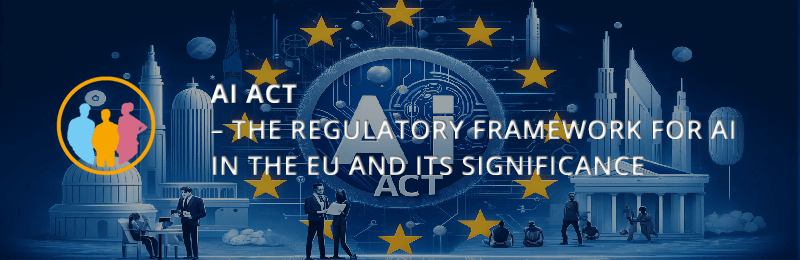
On May 21, 2024, the 27 EU member states adopted the AI Act, a comprehensive framework for regulating Artificial Intelligence (AI) within the European Union. This regulation is the world’s first comprehensive legal framework for AI, aiming to establish uniform standards and guidelines for the deployment of AI technologies. With the AI Act, the EU has laid a strong foundation for the regulation of artificial intelligence, promoting both trust and acceptance of the technology, as well as enabling innovations “made in Europe.”
The adoption of the AI Act by the EU Council is a significant step that will shape the future of artificial intelligence in Europe. The AI Act aims to maximize the benefits of AI while minimizing the risks. Through clear regulations and stringent requirements, it ensures that AI systems are deployed safely, transparently, and ethically.
In this blog post, we present the background and key contents of the AI Act, the specific provisions and their impact on innovation and the economy. We also highlight the national implementation in the member states and the international perspective of the AI Act.
Read moreBunny Business: Creative Social Media Ideas and Examples for your Businesses
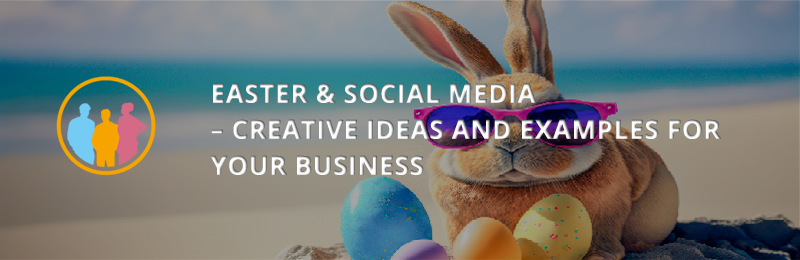
With Easter just around the corner, it’s time to start thinking about your social media strategy. Easter is a great opportunity for businesses to connect with their customers and build relationships. But what kind of content should you post? Here are some ideas and examples of Easter social media posts that will engage your audience and promote your business.
Read moreThe Significance of Customized Speech Commands Datasets in AI Training Strategies
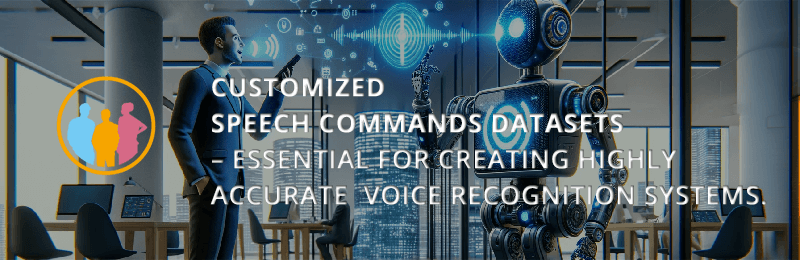
Have you noticed how AI is getting better at understanding us when we talk to our devices? It is all thanks to speech recognition technology. But to really make it work well, you as developers need to use customized speech commands datasets.
For example, think about when you are building a voice-controlled app. With a customized dataset, your app can understand specific commands better, like asking it to play a song or turn on the lights. It is like giving your app a superpower to understand fluent speech, context, and make the whole user experience smooth and intuitive.
These datasets, tailored to specific applications and domains, are crucial in shaping the training strategies of AI systems, particularly in automatic speech recognition (ASR) and voice-controlled applications.
In this blog post, we will delve into the importance of using customized datasets designed for specific applications, and explore how personalized speech datasets contribute to more accurate, reliable, and context-aware AI models.
Read moreIn-Store Audits: Improve Retail Performance with Crowd-Sourced Insights
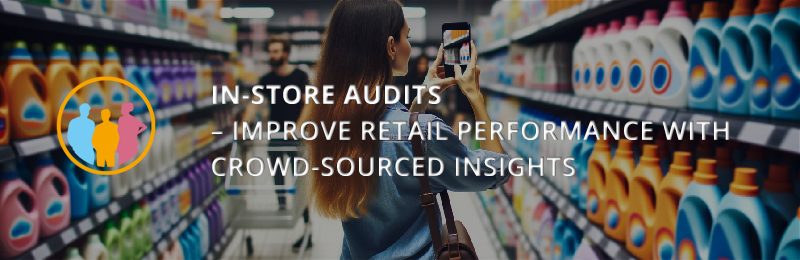
Optimizing retail performance is an ongoing challenge that requires strategic precision and innovative approaches. One critical aspect that can significantly influence success in retail is the efficacy of in-store audits. These audits are essential for ensuring products are attractively displayed, adequately stocked, and within convenient reach for shoppers. Utilizing crowd-sourced insights for in-store audits presents an opportunity to gather vast amounts of data efficiently and cost-effectively. This blog post delves into the mechanics of in-store audits and how crowd-sourced intelligence can refine and elevate the retail experience.
Read moreIncrease Your Productivity with AI Copilots
Welcome to the era of Generative Artificial Intelligence (Gen AI)! The buzz around this groundbreaking technology is contagious. It is accessible and gearing up to reshape organizations and the economy in ways that promise anything but dullness over the next decade.
According to McKinsey research, Gen AI is poised to automate 70% of business activities across various occupations by 2030, contributing trillions of dollars in value to the global economy.
Notably, the latest Gen AI application — AI Copilot, is garnering headlines for radically transforming the way businesses work amidst the complexities of digital modernization.
Much like digital Swiss Army knives, AI Copilots are adept at tasks ranging from boosting operational efficiency and aiding decision-making to fortifying security measures, simplifying content creation, and navigating intricate B2B sales processes.
Their versatility can be almost magical, leaving many intrigued about how to leverage this cutting-edge technology.
In this post, we’ll guide you through understanding AI Copilots and provide examples of how you can use them to unlock new levels of productivity and efficiency.
Read moreLLM Training: Strategies for Efficient Language Model Development
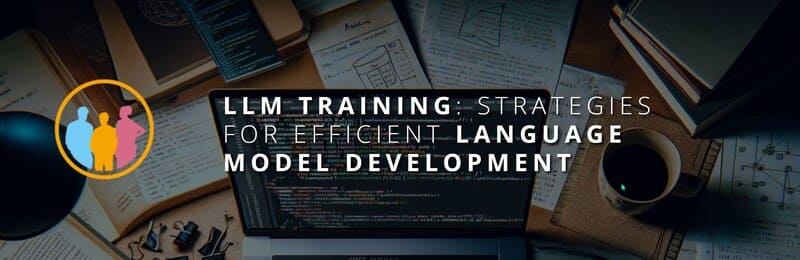
Content creation has been changed by large language models (LLM). These advanced machine learning architectures harness the power of vast amounts of textual data to perform a range of tasks under the umbrella of Natural Language Processing (NLP).
The training of LLMs involves meticulously structuring neural networks to generate human-like text, manage conversation, and even translate languages with remarkable accuracy.
Generative AI models, a subset of LLMs, are leading a paradigm shift in the way we interact with technology. Through training techniques that involve reinforcement from human feedback and innovations in model architectures, they have become central to developing AI systems that can comprehend and produce language effectively.
From streamlining customer service to powering virtual assistants, the applications of LLM are diverse, continuously expanding into new domains.
Their growing capabilities, however, come with a need for thoughtful consideration of ethical implications and the safety of AI systems. Ensuring that LLMs are trained to recognize and avoid harmful biases, respect user privacy, and make decisions transparently is critical for their responsible deployment.
Read more10 Ways Technology can Benefit your Growing Business
Technology serves as a catalyst for business growth, offering a myriad of opportunities for organizations to streamline operations, enhance productivity, and drive innovation. As businesses continue to evolve and expand, leveraging the power of technology becomes increasingly critical for staying competitive in a dynamic marketplace.
From improved efficiency and enhanced communication to access to global markets and better data management, the benefits of technology are vast and varied for growing businesses. In this article, we’ll explore ways in which technology can benefit your growing business, providing insights into how strategic adoption of technology solutions can fuel sustainable growth and success.
Read moreHarnessing the Power of AI in Cybersecurity: The Future of Digital Defense
The evolution of cyber threats has called for an effective threat detection and prevention system in cybersecurity.
Enter AI.
Previously, cybersecurity used signature-based detection to identify threats and malicious activities. While effective, this system required the antivirus software to recognize the threat and it also relied significantly on manual analysis.
Machine learning algorithms have facilitated companies to detect new and unknown threats without the need for human intervention. AI has caused a major shift in how businesses approach cybersecurity and allowed them to look for advanced ways in which they can safeguard their data and systems.
Read more