Image annotation and artificial intelligence
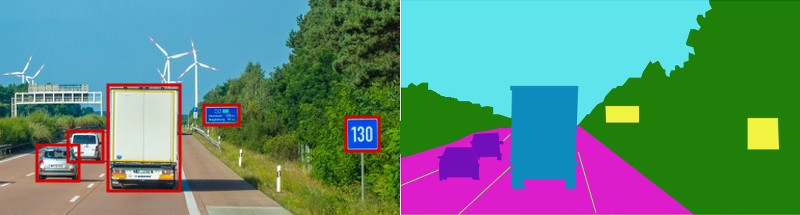
Everyone has heard something about artificial intelligence. However, the term image annotation is less common. Image annotation describes the classification of information that is of relevance to an image. Recognizing the content of images is an important factor for many automatized processes. In order for machines to capture the meaning and individual components of images, artificial intelligence is required, in which a human-like analysis of images is simulated. To achieve this, countless training data in terms of human input are required.
Recognizing the meaning of images
In the course of evolution, human beings learned how to grasp sensory input with their intelligence. This is why it is fairly simple for us to understand the content of an image:
- What does the detail in an image signify?
- Where are specific people located in a street scene?
- In which respect do the different images resemble each other?
These are just a few of the typical questions image annotation focuses on. Digital systems have been used to provide answers for a long time. Machines can read images – but often, very cumbersome programming work is needed to recognize the content of still and moving images. And the more complex the task is, the more one has recourse to artificial intelligence, i.e. to programs that are capable of learning. The services of crowdworkers are suitable for creating a foundation for artificial intelligence within the context of image annotation.
Machines also need to be trained
How can one train a program that enables automatic image annotation and functions according to the principles of artificial intelligence? The prerequisite for the training is a large number of images, which are initially processed by human beings. Artificial intelligence uses these commented images as templates.
For example: The task of the user is to mark typical street scenes. This is done by marking various image objects on the screen in different colors. In doing so, every traffic light, every traffic sign, every vehicle and every pedestrian is given a color. This processed image is then communicated to the program. By comparing the similitudes and differences, the software gradually recognizes what typical visual features the respective image details have. The program therefore also recognizes which objects are relevant and which ones are not – depending on the purpose of the program.
Tip:
Do you need support with the annotation of images? clickworker offers you the proper solution.
Learn more about our
Image Annotation Services
Image annotation tools
The various annotation methods depend on the complexity of the visual templates. Clearly definable and eye-catching road lines can be easily marked one-dimensionally. Other, non-linear objects are made visible with so-called bounding boxes. This is done by using colored frames to mark people or traffic signs. The next step is the cubes. These three-dimensional frames are used to capture the spatial structure of objects. To be more exact, training uses full pixel segmentation.
It goes without saying that the more exact the image annotation method employed is, the higher the computational cost (the complexity of the algorithms) will be. The three-dimensional markings using cubes or full segmentation will for instance be put to use in programs that are used for self-driving vehicles or drones.
Machines learn image interpretation
To be able to obtain an exact and reliable awareness of the environment, machines initially need the achievements and results provided by human beings.
Ultimately, the closer an artificial intelligence for image recognition comes to the human interpretation of images, the better it is.
More training data, as an input for the programs, usually leads to a higher quality of the results and a lower error rate.
Crowdsourcing is a perfect way of achieving exactly this high input.
The type and scale of the data to be delivered and/or processed is basically unlimited and depends on the needs of the developers and the training methods used.
Crowdsourcing ensures the human input
Many image annotations can be carried out within a very short amount of time with the help of crowdsourcing. This is also because these tasks are very popular among the crowd. To attract high-performance teams for the processing of particular tasks, the participants must take qualification tests, thus ensuring the quality of the work results. Leading providers of crowdsourcing solutions such as clickworker offer their own electronicadvanced image annotation tool that help the customer save additional time and effort. This significantly accelerates the automatization of visual systems. Crowdworkers make a decisive contribution to the quality of artificial intelligence for image annotation.