The Ultimate Guide to Machine Learning in Finance: Revolutionizing the Future of Financial Institutions
Author
Robert Koch
I write about AI, SEO, Tech, and Innovation. Led by curiosity, I stay ahead of AI advancements. I aim for clarity and understand the necessity of change, taking guidance from Shaw: 'Progress is impossible without change,' and living by Welch's words: 'Change before you have to'.
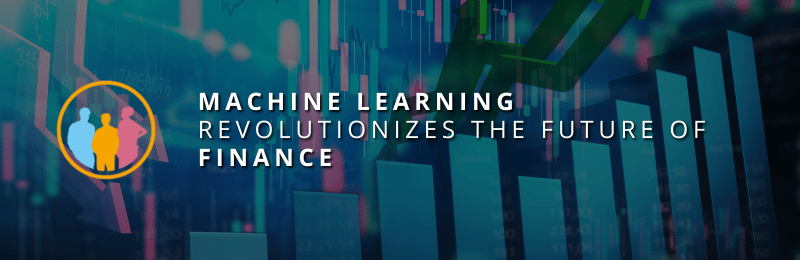
Machine learning (ML) has revolutionized many industries, and finance is no exception. With the massive amount of data available in finance, you can use machine learning algorithms to analyze, predict, and optimize various financial processes. From fraud detection and risk management to automated trading and tax optimization, machine learning has a broad range of applications in finance.
The post will talk about how machine learning is changing the face of finance and the benefits it offers. We will delve into some specific use cases where machine learning is applied in finance and discuss the implications and challenges of this technology.
Table of Contents
What is Machine Learning in Finance?
ML in finance involves using algorithms and statistical models to analyze large amounts of financial data and generate insights without explicit instructions. It is a subset of AI technology that creates new logs based on updated data. In finance, machine learning is used to automate financial processes, analyze data, and detect patterns that would be difficult for humans to identify.
One of the most significant advantages of machine learning in finance is data evaluation with accuracy. Financial institutions generate an enormous amount of data daily, including market, customer, and transactional data. Machine learning algorithms can process this data and generate insights that would be impossible to identify manually. This can lead to better risk management, more informed investment decisions, and improved customer service.
Machine learning has many applications in finance, including fraud detection, automated trading, algorithmic trading, anomaly detection, robo-advisors, and tax optimization. With fraud detection, machine learning algorithms can detect unusual patterns in financial transactions that indicate fraudulent activity. Machine learning algorithms help with patterns and trend recognition in automated trading by analyzing market data and executing trades to optimize trading strategies.
Machine learning is transforming the finance industry by providing new ways to analyze data and make decisions. As the technology continues to evolve, we expect to see even more innovative applications of machine learning in finance.
Benefits of Machine Learning in Finance
There are numerous benefits to using ML in finance. One of the most significant advantages is processing and analyzing raw data and creating valuable insights. Financial institutions generate an enormous amount of data daily, including market, customer, and transactional data. Machine learning algorithms can process this data and generate insights that would be impossible to identify manually. This can lead to better risk management, more informed investment decisions, and improved customer service.
Another significant benefit of ML in finance is its ability to automate processes. Machine learning algorithms can automate various financial processes, such as fraud detection, anomaly detection, and tax optimization. It reduces the risk of fraud and error while saving time.
Machine learning can also improve decision-making in finance. Machine learning algorithms can make predictions and inform decision-making by analyzing historical data and identifying patterns. This is especially useful in trading, where machine learning can be used to develop trading strategies and execute trades automatically.
Another area where machine learning is beneficial in finance is customer service. Robo-advisors, powered by machine learning algorithms, can provide personalized investment advice and help customers manage their finances, and it improves customer satisfaction and reduces the workload of financial advisors.
Overall, the benefits of ML in finance are vast. Machine learning transforms the finance industry from improving decision-making and automating processes to providing better customer service and identifying new opportunities. We expect to see even more innovative machine-learning applications in finance, and financial institutions that embrace this technology will be better positioned to succeed.
Drawbacks of Machine Learning in Finance
While ML has many benefits in finance, it also has some drawbacks that you must consider. The major drawback is the potential for bias in the algorithms. Machine learning algorithms use historical data, which may contain bias, leading to biased predictions or decisions. For example, a machine learning algorithm trained on historical data that contains gender bias may make biased loan approval decisions.
Another potential drawback of ML in finance is the lack of transparency. Machine learning algorithms can be complex because it gives insight that experts can usually set and understand only. This can make identifying and correcting errors or biases in the algorithms challenging.
Another potential issue is the lack of human oversight. While machine learning algorithms can automate many financial processes, they may not be able to detect unusual situations or make decisions based on context. This can lead to errors or incorrect decisions, especially in situations not covered by the algorithm’s training data.
There is also a risk that machine learning algorithms may be vulnerable to cyber-attacks. Hackers may attempt to manipulate or deceive the algorithms to access sensitive financial data or disrupt financial markets.
Finally, there is the issue of the cost of implementing machine learning in finance. Developing and maintaining machine learning algorithms requires significant time, money, and resources. Therefore, small businesses might find it difficult to integrate into their business, limiting their ability to compete with larger firms with the resources to invest in machine learning technology.
Besides benefits, machine learning in finance has concerns and drawbacks as well. By addressing these concerns and implementing appropriate safeguards, financial institutions can leverage the benefits of machine learning while minimizing the risks.
Types of Machine Learning in Finance
Machine learning has become increasingly prevalent in the finance industry, offering many benefits ranging from automated trading to fraud detection. Several types of machine learning are commonly used in finance, including supervised, unsupervised, and reinforcement learning.
A machine learning algorithm trains a model with the labeled dataset. Supervised learning helps with fraud detection, credit risk analysis, and stock price prediction. For example, a supervised learning algorithm could be trained to identify fraudulent transactions based on historical data labeled as fraudulent or legitimate.
Unsupervised learning is a model trained with an unlabeled dataset. Unsupervised learning is often used in finance for anomaly detection and clustering tasks. For example, an unsupervised learning algorithm could identify unusual trading patterns in financial markets.
Reinforcement learning is models that generate data through trial and error. In finance, reinforcement learning is often used for automated trading and portfolio optimization tasks. For example, a reinforcement learning algorithm could be used to develop a trading strategy that maximizes returns while minimizing risk.
4. Gaussian Processes
Gaussian processes are a machine learning algorithm commonly used in finance for portfolio optimization and risk management tasks. Gaussian processes model the distribution of possible outcomes for a given set of inputs, allowing financial institutions to make more informed decisions based on the uncertainty of the data.
5. Neural Networks
Neural networks have a similar model to the human brain. In finance, neural networks are often used to predict stock prices and detect fraud. For instance, you can train a neural network to identify fraudulent transactions based on patterns in the data.
6. Deep Learning
Deep learning uses neural networks to model a model with complex data, and you can add multiple layers of neural networks. In finance, deep learning is often used for sentiment analysis and natural language processing tasks. For instance, you can analyze news articles and social media posts to predict market trends.
Several types of machine learning are commonly used in finance, each with strengths and weaknesses. By understanding these different types of machine learning, financial institutions can choose the best approach for their specific use case.
When to Use Machine Learning in Finance?
Machine learning solves various financial institution problems, but using it appropriately and judiciously is important. Here are some scenarios where machine learning may be particularly useful in finance:
- Large Datasets
The major benefit of machine learning is quick and accurate data analysis, regardless of size. If a financial institution has a large dataset that would be difficult to analyze manually, it can use machine learning to identify patterns and generate insights.
- Complex Data
Machine learning can be particularly useful for analyzing complex data, such as natural language processing or sentiment analysis. In these cases, traditional methods won’t help you identify data problems.
- Predictive Analytics
Machine learning is often used for predictive analytics, such as predicting stock prices or credit risk. By analyzing historical data, machine learning algorithms create a pattern and identify trends. Using these patterns, teams can make informed predictions and decisions.
- Automation
Machine learning can also automate processes, such as fraud detection or portfolio optimization. By automating these processes, financial institutions can save time and resources while improving accuracy and efficiency. (Excursus: Automation Paradox)
However, it doesn’t resolve all financial problems, and it is important to carefully consider the problem and determine whether machine learning is the best approach. Moreover, the data used to train machine learning algorithms is accurate, relevant, and unbiased.
Quick Tips for Machine Learning in Finance
- It is important to clearly define the problem you are trying to solve with machine learning and determine if it is appropriate for the task.
- There are many machine learning algorithms, and choosing the right one for your problem is key.
- Machine learning algorithms require relevant and accurate data, so ensuring that your data is high quality is important.
- Machine learning models are not static and may need to be updated over time, and it is important to monitor their performance and update them as necessary.
- Machine learning can introduce ethical considerations, such as privacy and bias. Considering these implications and ensuring that your use of machine learning is ethical and responsible is important.
By following these tips, financial institutions can effectively and responsibly use machine learning to improve their operations and better serve their clients.
Conclusion
Machine learning transforms financial institutions’ operations, from fraud detection to portfolio optimization. By leveraging large datasets and complex algorithms, financial institutions can gain valuable insights and make informed decisions that benefit their clients and their bottom line.
However, you should consider the potential drawbacks of machine learning and ensure that it is used responsibly and ethically. With the right approach, financial institutions can harness the power of machine learning to improve their operations, enhance customer experiences, and drive innovation in the industry.
FAQs on Machine Learning in Finance
What is the difference between machine learning and traditional methods in finance?
Traditional methods in finance typically involve manual analysis of data and the use of pre-defined rules and models to make decisions. Machine learning, on the other hand, uses algorithms to identify patterns and connections in large datasets automatically. Machine learning can be particularly useful for analyzing complex data and making predictions.
How is ML used in finance?
Machine learning is used in finance for various tasks, including fraud detection, automated trading, anomaly detection, robo-advisors, tax optimization, risk management, and more. Machine learning algorithms can help financial institutions to analyze large amounts of data and identify patterns and trends.
What are some potential drawbacks of ML in finance?
One potential drawback of machine learning in finance is the potential for bias in the data or algorithms used. For new users, the models can be complex. Finally, there is always the risk of errors or inaccuracies in the data or algorithms used, which can have serious consequences in the financial industry.
How can financial institutions ensure that their use of machine learning is ethical and responsible?
To ensure that their use of machine learning is ethical and responsible, financial institutions should carefully consider the potential biases and implications of the data and algorithms used. It is important to regularly monitor the performance of machine learning models and update them as necessary to ensure accuracy and relevance. Additionally, financial institutions should consider the ethical implications of using machine learning and ensure that it aligns with their values and mission.
What are some emerging trends in ML in finance?
Some emerging trends in machine learning in finance include using neural networks, deep learning, and reinforcement learning for more advanced and complex tasks. Natural language processing (NLP) is also used to analyze text data and improve sentiment analysis and other tasks. Finally, Gaussian processes are being explored for their potential to improve risk management and decision-making in finance.