Harnessing Machine Learning for a Revolution in the Insurance Industry
Author
Robert Koch
I write about AI, SEO, Tech, and Innovation. Led by curiosity, I stay ahead of AI advancements. I aim for clarity and understand the necessity of change, taking guidance from Shaw: 'Progress is impossible without change,' and living by Welch's words: 'Change before you have to'.
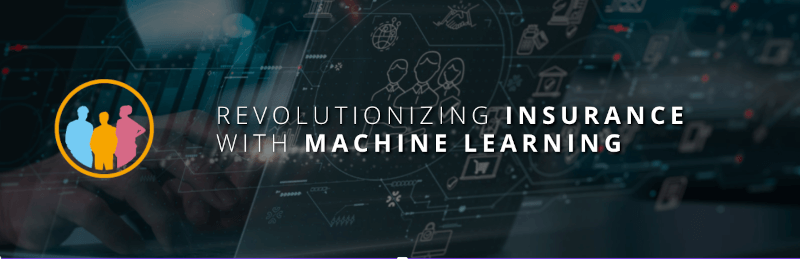
Have you ever wondered about the future of the insurance industry? It’s not as far off as you might think. In fact, it’s on the brink of a significant transformation, one that’s being driven by the power of machine learning. This technology, with its capacity to handle and learn from vast data, is poised to revolutionize the industry.
From improved risk assessment and fraud detection to enhanced claims management and customer experience, the potential benefits are immense. As we delve into this topic, we’ll explore how machine learning is reshaping the insurance industry and the exciting possibilities it holds for the future.
Table of Contents – Machine Learning in Insurance
Machine Learning and Risk Assessment
Risk assessment – the heartbeat of the insurance industry – has been traditionally steeped in manual processes and human judgment. But with machine learning, it’s undergoing a sea change.
How Machine Learning Works in Risk Assessment
Machine learning uses complex algorithms that can learn and improve through exposure to data. In risk assessment, these algorithms process large volumes of data, identifying patterns and correlations that might not be readily visible to human analysts. This leads to more accurate predictions of risk factors, enabling insurers to make better-informed decisions.
- Improved Accuracy: Machine learning algorithms can handle vast and diverse data sets, leading to more precise risk predictions.
- Enhanced Speed: They can process data and deliver insights significantly faster than traditional methods.
- Greater Efficiency: By automating the risk assessment process, insurers can save time and resources.
Benefits for Insurers and Insured
Machine learning in risk assessment provides benefits to both insurers and their customers:
- Insurers: Machine learning allows insurers to accurately price their policies according to the individual’s risk level, minimizing financial risks and enhancing profitability.
- Customers: Customers get access to more personalized offerings and fairer premiums, leading to improved satisfaction and trust in their insurers.
Example
The use of telematics in auto insurance is an excellent illustration of machine learning in risk assessment.
Here’s how it works:
- Insurers collect data from devices installed in vehicles.
- This data is analyzed using machine learning algorithms to assess driving behavior.
- Based on the analysis, the driver’s risk level is determined, affecting the premium they pay.
Fraud Detection Using Machine Learning
Insurance fraud is a significant drain on the industry, with estimates suggesting it costs the U.S. insurance industry over $40 billion (FBI.gov) per year, excluding health insurance.
Significance of Machine Learning in Fraud Detection
Machine learning comes into play as a potent tool to detect fraudulent activities. It scrutinizes claims history, customer information, and other relevant data, identifying suspicious patterns that might signify a fraudulent claim.
Traditional Methods | Machine Learning |
---|
Manual and time-consuming | Fast and automated |
May miss complex patterns | Excels at pattern recognition |
High potential for human error | Reduced errors due to automation |
Impact on the Insurance Industry
The adoption of machine learning in fraud detection can save insurers substantial amounts in fraudulent claim payouts, increasing profitability and instilling a sense of fairness within the insurance ecosystem.
Real-World Example
Anadolu Sigorta, one of Turkey’s largest insurers, is an example of a company that uses machine learning for fraud detection. Before implementing the technology, the insurer had a team of 50 people manually checking every claim using a set of loose rules and their personal experience. However, after switching to a predictive analytics tool with AI/ML-based software, the insurer was able to speed up the process and reduce errors in real-time risk assessment. As a result, Anadolu Sigorta saw a 210% ROI within one year of implementing the AI solution. This example shows the potential benefits of using machine learning in insurance fraud detection, including reducing risks and costs related to fraud and achieving superior results.
Machine Learning in Claims Management
Machine learning facilitates faster and more accurate claims processing, making the process smoother for both insurers and claimants. From assessing claim validity to determining the payout amount, machine learning can streamline every step.
Role of Machine Learning in Claims Management
Machine learning facilitates faster and more accurate claims processing, making the process smoother for both insurers and claimants. From assessing claim validity to determining the payout amount, machine learning can streamline every step.
Traditional Claims Management | Machine Learning-Enabled Management |
---|
Time-consuming and manual | Quick and automated |
Potential for human errors | Reduced errors due to automation |
Inconsistent claim assessment | Consistent and accurate claim assessment |
Real-World Example
Fukoku Mutual Life is a prime example of how machine learning can be used in claims management. The Japanese insurance company utilizes AI and deep learning to handle claims data, which enables it to automatically access and analyze medical documents related to the case, as well as calculate payouts. By automating these processes, Fukoku Mutual Life has achieved a 30% increase in productivity and cost savings of around $1 million per year. This is a testament to how machine learning can help insurers to streamline claims management, improve decision-making, and ultimately provide better service to their customers.
Personalized Customer Experience
In an age where customers expect personalized experiences, machine learning can help insurers meet these expectations.
How Machine Learning Enhances Customer Experience
Machine learning can analyze vast amounts of customer data, from demographic information to past interactions, to understand individual preferences and needs. Insurers can use these insights to offer tailored products, personalized communication, and more efficient service.
- Tailored Products: Insurers can use machine learning to understand customer needs and offer products that fit those needs precisely.
- Personalized Communication: Machine learning can help insurers communicate with customers in a way that resonates with them, improving engagement and satisfaction.
- Efficient Service: Machine learning can automate many aspects of customer service, resulting in quicker response times and higher customer satisfaction.
Example
Many insurers are harnessing machine learning to provide personalized experiences. For instance, some are using recommendation engines to suggest suitable insurance products based on the customer’s profile and history.
Enhanced Decision Making with Predictive Analytics
In the fast-paced world of insurance, making informed decisions quickly is paramount. That’s where predictive analytics comes in. This sophisticated technology, fuelled by the power of machine learning, is helping insurance companies to anticipate future trends and events based on historical data, allowing them to make proactive, data-driven decisions.
Role of Machine Learning in Predictive Analytics
Predictive analytics, powered by machine learning, has become an essential tool for insurers. By analyzing historical data, machine learning can forecast future trends and events, helping insurers make more informed decisions.
Here are a few ways predictive analytics can revolutionize the insurance industry:
- Pricing Policies: Predictive analytics can help insurers better understand the risk associated with a particular policy, enabling them to price it accurately.
- Customer Retention: By identifying patterns in customer behavior, predictive analytics can predict which customers are likely to churn, allowing insurers to proactively address their concerns.
- Marketing and Sales: Insurers can use predictive analytics to identify which products are likely to appeal to which customers, leading to more effective marketing and sales strategies.
Real-World Example
One of the most significant insurance companies that use machine learning in predictive analytics is Progressive. This US-based corporation specializes in vehicle insurance and has implemented ML algorithms to predict car accident possibilities for potential customers. They have created a mobile app and a plugin device for vehicles called Snapshot that gathers driving data and uses it for further predictive analysis. Based on the analytics, Progressive attracts and builds long-term relationships with reliable and careful drivers. With the help of machine learning, Progressive has been able to reduce the risk of accidents and provide better insurance rates to its customers. Their use of machine learning in predictive analytics has allowed them to stay ahead in the competitive insurance industry.
The Power of Natural Language Processing in Insurance
Natural Language Processing (NLP), a subfield of machine learning focused on the interaction between computers and human language, has significant potential in the insurance industry.
Role of NLP in Insurance
NLP can analyze text data from a variety of sources, such as customer emails, social media posts, and claim documents, to extract valuable insights. These insights can inform everything from customer service to fraud detection.
- Improved Customer Service: NLP-powered chatbots can handle customer queries, provide information, and even guide customers through the claims process.
- Fraud Detection: By analyzing the language used in claims, NLP can help identify fraudulent claims.
- Sentiment Analysis: NLP can assess customer sentiment from online reviews and social media posts, helping insurers improve their offerings and communication.
Real-World Example
Manulife is a Canadian-based insurer that uses machine learning for natural language processing to improve customer service. Manulife handles data of over 26 million clients from all around the world and has begun a four-year cooperation with Waterloo Artificial Intelligence Institute to implement predictive models for disability claims, fraud detection, and natural language processing. With NLP, Manulife can analyze and understand customer inquiries and feedback to provide personalized and efficient responses. This technology helps the company to improve customer satisfaction and experience, which is a crucial factor in retaining clients in the insurance industry. Overall, Manulife’s use of NLP demonstrates the potential of machine learning in enhancing customer service and building long-term relationships with clients.
AI and ML in Underwriting
Underwriting, a crucial process in insurance, involves evaluating a customer’s risk profile to determine the terms of coverage. This process, traditionally manual and relying heavily on human judgment, is being transformed by the power of artificial intelligence (AI) and machine learning.
The Traditional Underwriting Process and Its Challenges
Traditionally, underwriters would manually evaluate risk by reviewing a range of data such as credit scores, claims histories, medical records, and more. This process, while effective, was time-consuming and prone to human error. In addition, it often didn’t account for a broad range of data, which could lead to inaccurate risk assessments.
How AI and Machine Learning Revolutionize Underwriting
AI and machine learning can analyze vast amounts of data much more quickly and accurately than a human can. This includes not just traditional data like credit scores and claims histories, but also non-traditional data sources such as social media posts and online behavior, providing a more comprehensive view of a customer’s risk profile.
Key Benefits:
- Efficiency: AI can quickly analyze a vast range of data, significantly speeding up the underwriting process. This means quicker policy approvals and a better customer experience.
- Accuracy: By considering a wider range of data, AI can make more accurate predictions about risk, leading to fairer pricing and better risk management.
- Consistency: AI ensures that the underwriting process is consistent, free from human bias or error.
Example
AI in underwriting isn’t a concept of the future; it’s happening now. Several insurance companies are already using AI to improve their underwriting processes. For instance, some are using AI to automate parts of the underwriting process, while others are using it to improve the accuracy of their risk assessments.
Future Insurance: The Next Frontier of Machine Learning
As we consider the future of insurance, it’s clear that machine learning and artificial intelligence will play increasingly significant roles. These technologies offer the potential to completely transform the industry, leading to more accurate risk assessments, more personalized coverage, and more efficient operations.
Predictive Modelling
One of the most exciting applications of machine learning in future insurance is in predictive modelling. By analyzing vast amounts of data, machine learning algorithms can forecast future events, trends, and behaviors. This capability could allow insurers to anticipate claims before they happen, leading to more proactive risk management.
Personalization
In the future, we may see more insurance policies tailored to the individual’s specific needs, behaviors, and risk profile. This kind of personalization, enabled by machine learning, could lead to fairer pricing and better customer satisfaction.
Automation
As machine learning algorithms become more sophisticated, we could see increased automation in the insurance industry. Tasks like claim processing, customer service, and even underwriting could be automated, leading to more efficient operations and quicker response times.
The Role of clickworker in Shaping Future Insurance
As we’ve seen, machine learning holds tremendous potential for the insurance industry. However, to fully leverage this potential, high-quality, diverse, and accurate data is essential – and that’s where clickworker comes in.
We provide a wide array of data services that can enhance the effectiveness of machine learning in insurance. From text creation and categorization to AI training data, clickworker can help insurers ensure their machine learning algorithms are fueled by the best possible data.
- AI Training Data: We provide a wide range of AI training data services, supporting insurers in various machine learning applications, from risk assessment to fraud detection.
- Data Collection and Annotation: clickworker can gather and annotate data, ensuring that machine learning models have the diverse, high-quality data they need.
- Text Creation: Our pool of qualified workers can create diverse text content, helping to train and refine machine learning models that handle text data, such as chatbots.
Final Word
In conclusion, the future of insurance is undeniably intertwined with machine learning and artificial intelligence. These technologies hold the potential to completely transform the industry, leading to more accurate risk assessments, more personalized coverage, and more efficient operations. But to fully leverage this potential, insurers need high-quality, diverse, and accurate data. That’s where clickworker comes in. We provide a wide array of data services that can enhance the effectiveness of machine learning in insurance. From text creation and categorization to AI training data, clickworker is here to ensure your machine learning algorithms are fueled by the best possible data. Ready to embrace the future of insurance? Get in touch with clickworker today.
FAQs on Machine Learning in the Insurance Industry
How is machine learning used in insurance?
Machine learning is used in insurance to help underwriters predict which customers are more likely to have an accident or make a claim. This allows insurers to set premiums that are better aligned with the risk of the customer, which can help to make insurance more affordable.
How AI and machine learning are used to transform the insurance industry?
AI and machine learning can help insurance companies to better assess risk, identify fraud, and automate repetitive tasks. This can result in lower premiums for customers and increased efficiency for the company.
Do insurance companies use algorithms?
Yes, insurance companies use algorithms to help determine premiums and coverage. Algorithms are used to evaluate risk and calculate potential losses.