Text classification – areas of application on the Internet
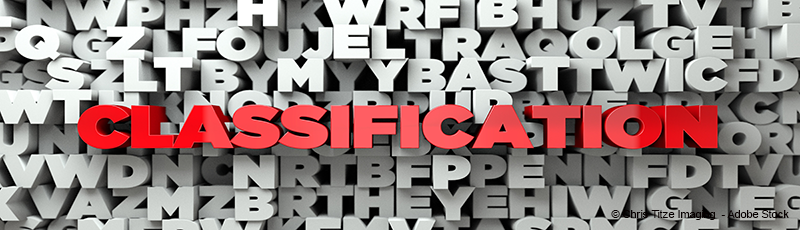
There are billions of websites with countless texts on the Internet. This makes it difficult to keep track of them. Text classification is a method that provides an overall view and structures the offer. Which application areas are there for text classifications in the World Wide Web?
Cleaning up on the Internet
The amount of data on the Internet is so large that filtering by human experts alone is impossible to conceive. The more information is spread on the Internet, mainly in text form, the greater the need for machine analysis, sorting and classification. Examples:
- News portals select their news according to subject areas and other features. A human being preferably makes the final decision as to whether and where a source should be placed in a portal – but artificial intelligence can also perform this task mechanically.
- Vertical search engines only capture links to a specific topic – in contrast to universal search engines such as Google or Bing. Vertical search engines advertise with the advantage that they make it easier for interested users to find relevant information faster. This is because the index is limited from the outset to topic-specific content.
- Email provider need efficient procedures to differentiate between legitimate messages and spam mails using various criteria. These criteria include not only the sender, but also the text itself. Spam mails are characterized by typical linguistic characteristics.
- Sentiment analyses are used for market research. These algorithms are used to automatically detect positive or negative attitudes – for example regarding certain products or ongoing campaigns.
Machine support is an effective aid for classifying texts. Artificial intelligence plays an increasingly important role here.
Text classification and machine learning
Artificial intelligence shows that it is also useful in the classification of texts. In this case, the knowledge acquisition of the algorithms is based on training data that are already pre-classified. New text documents are gradually compared with these training data. The principle of trial and error provides increasingly accurate results.
The problem with the analysis of words lies mostly in filtering out the irrelevant features. One approach for this is so-called stemming – each word is systematically traced back to the root of the word. By excluding superfluous features, the runtime of the programs is considerably reduced.
When classifying texts, not the meaning of individual words ultimately matters, but the context in which they are used.
For example: Even if the word flower does not appear in a text, the text nevertheless deals with the topic if words relating to the environment are used frequently, for example roses, tulips, garden or fertilizer.
Tip:
Let clickworker process text classification via their crowd to
obtain high-quality training data for your AI system.
Obviously, any machine text classification has a certain probability of errors. The higher the probability of an appropriate classification, the better the underlying algorithm.
Complexity
The complexity of a text document is an important factor for the classification of documents. How complex is a text? There are some indications. These are for example:
- The average word length,
- The average number of words in a sentence
- And the type token relation (ratio of total words to the number of different words).
The classification of texts in terms of complexity offers added value in particular for Internet portals, which provide their visitors with a target group-specific range of links. The text classification also helps meeting different requirements, for example with regard to
- the intellectual aspiration of a text,
- the degree of focus on a particular sub-theme (as opposed to comprehensive representations),
- or the classification of texts in terms of reading time.
In this respect, text classification is an efficient means of preserving the coherent style of a portal even when integrating external sources.
Detecting tendencies in advance: Sentiment analysis
An important application of text classification is sentiment analysis. Sentiment analysis is a sub-area of text mining.
Text mining uses algorithms to filter out the core information from unstructured texts. In the (utopian) ideal case, this type of algorithm represents the intellectual process of human reading.
A sentiment analysis reveals whether a text (e.g. an evaluation comment or a post in social networks) has an overall positive or negative basic tendency – solely on the basis of what is written, regardless of any points or stars awarded. It is difficult to highlight this mood of a text because a document as a whole can contain both positive and negative statements. However, one can determine the text’s overall tendency relatively accurately by statistical and linguistic means.
Sentiment analyses in marketing
Sentiment analyses are particularly suitable for marketing purposes to get opinions about ongoing campaigns in order to be able to react unerringly to them.
- Which advertising measures go down well with the customer and which do not?
- How do current company developments affect the company’s reputation?
- Are there any noticeable changes in the perception of relevant moods?
Text classification is a good way to understand the target group’s language – and making use of it for marketing purposes. No company can afford not to speak the same language as its customers.
Summary
The advantages of automatic text classification are obvious and they increase as the amount of information on the Internet expands. An additional push factor for text classification services is the fact that companies must always have an overview of any developments that are relevant to the market and that are emerging as trends on the web.