Choosing the right AI model
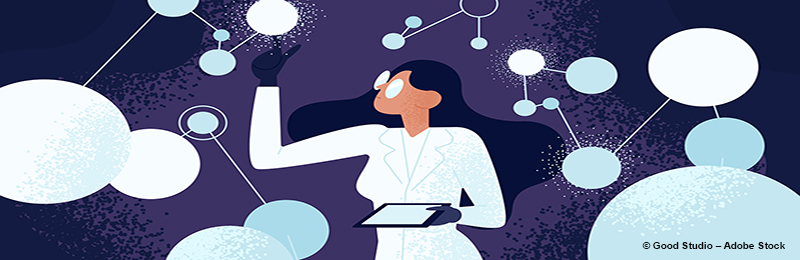
The popularity of AI (Artificial Intelligence) systems is growing in leaps and bounds. Businesses around the world are starting to understand how these systems can benefit them, but AI is not yet at the stage where you can simply take it out of the box and expect it to perform. AI systems require training, which is where AI models come into the equation.
An “AI model” is an algorithm that is taught using data and input from a human expert. The model is evaluated on its accuracy by comparing the results it provides against the decisions made by the human expert. This often requires the algorithm to review a significant amount of data. In addition, by accessing data from multiple sources, the model is better able to find patterns which it can use to further streamline its analysis. The model is attempting to replicate the decision process of a team of experts with access to the same data.
What Model is the Right Model?
When considering models for AI, often there is more than one right answer. There are many different models that can be looked at and some of these algorithms are better than others, based on the problem being analyzed. For example, with AI builder, using an object detection model is vastly different to a prediction model. In each case, how they work, the information they are looking for, and the results they provide will vary based on the models.
Prediction Model
With a prediction model, you are looking for yes/no patterns that your data presents. Tools like AI builder can use the information from past results to learn and predict future results. This type of model is great for businesses looking for yes/no, pass/fail type responses. For example, trying to understand what accounts might not pay on time or which customers are most likely to renew their service.
With a prediction model, it is best if the person creating the model understands the business and what the data being analyzed means. As a case in point, if you are trying to understand customer churn you would need to ensure that the data being analyzed correctly indicates if a customer has churned or not and where this information is stored. With this information to hand, the model can be trained to look for patterns where churned customers were influenced by other decisions.
Category Classification Model
Data of all types is increasing in volume, scope, and complexity. The source of data – its channels – is similarly increasing in size with email, text, social media and others contributing even more massive amounts of information. While data has valuable information and insights, obtaining the right information in this ocean is difficult and time-consuming.
Category classification addresses this issue through tags that can be used to sort data based on spam, sentiment, customer requests and other themes. By using category classification models, companies are better able to automate and scale business processes. With category classification models, it is important to ensure that tags are balanced in their use in the training data and similarly, the data being used should match the expected data as much as possible.
Entity Extraction Model
With entity extraction, you are actually pulling specific information from a larger data set. The entity extraction model looks for specific data contained in a set of text based on your organization’s needs. This helps to transform unstructured data into structured machine-readable data.
Form Processing Model
The form processing model is able to extract key information from your online forms. The forms themselves can be in a variety of different formats from image files (JPG, PNG) to PDF and a minimum of five forms is required to start training the model.
Object Detection Model
With object detection, you are looking at images. This type of model requires a significant volume of images for each data set. If not enough images are available, the model might not be able to correctly identify relevant similarities. In addition, it is important to ensure that you have a balanced volume of images – hundreds of one type and only 5 or 10 of another can skew the data.
Order a large volume of photo or image datasets specifically created by Clickworkers for your model to train your AI system optimally.
With the object detection model, the data should be as diverse as possible. Pictures of cats (if that is the subject) should not only show cats on the sofa but in multiple locations and poses to make the model the most effective. Consider criteria such as lighting, background, size and photo angles as each of these plays a factor. Also look for negative bias where the AI model focuses on another common factor in the photo instead of the item you are interested in.
Choosing the Right Model
Choosing the right model depends on your business case and requirements. Some key factors to consider include the Volume, Variety and Velocity of Input of the data you want to process. This is known as the 3V’s of Big Data. In addition, you need to know how much time you can spend on data processing as well as the amount of resources you have available. Perhaps most importantly though, it is critical to know the goal you are attempting to achieve with your data processing.
AI Models in the Real World
Investments worldwide in AI models continue to grow. Models have been used across a host of industries and sectors and while they have shown great promise in some areas, while in others they are still lacking. On the online front, social media, image and speech recognition for example, AI models are meeting and exceeding their creator’s goals and intentions.
In other sectors however, they are being impacted by dynamic factors like changing weather conditions that were not considered or accounted for within the model. At the current time, this gap can only be addressed by onsite human intervention, however as data increases in scope, size and detail, models will continue to grow in skills and expertise.
AI models are not something that can simply be created and left alone. Companies are consistently changing based on changing business needs and macroeconomic trends. These changes impact the products and processes within an organization and AI systems also need to be able to adapt to these changes.